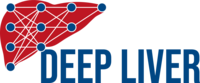
What we do
We pursue four specific objectives:
1.) The development of deep learning methods for diagnosis and risk prediction of liver diseases based on histological image data, including multicentre blinded validation:
Using a subset of samples, deep learning systems will be developed that automatically pre-process histological image data from routine liver biopsies and perform quality control and colour normalisation and generate small, overlapping image areas ("tiles") with which a deep neural network will be trained. This Image DeepNet will be trained to diagnose the patient's disease (including NAFLD, PBC, PSC) and predict the clinical course. This diagnostic tool will be validated in a blinded fashion using large, multicentre patient cohorts.
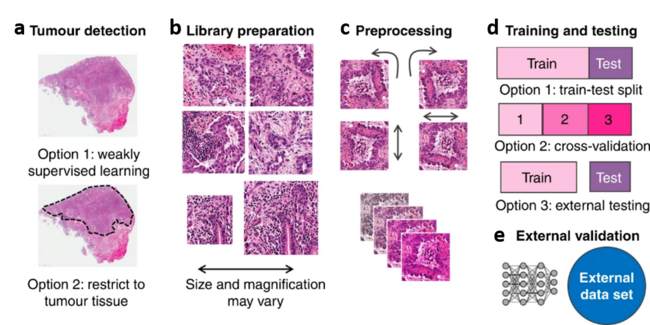
2.) Systematic comparison of image-based risk prediction with clinical data and classical risk scores as well as development of multimodal deep learning systems:
Deep learning networks will be trained on routine clinical data to diagnose and predict clinical endpoints (Clinical DeepNet). The performance of this methodology will be compared with pure image-based data analysis. Subsequently, the information from the Clinical DeepNet will be combined with the Image DeepNet and summarised using classical machine learning models.
3.) Explaining statistically predictive features and finding novel clusters and previously unknown biological subgroups of liver diseases based on unsupervised methods.
In a "reverse engineering" step, those features in the data set are to be identified which are particularly associated with the target variable. In image data, this could be, for example, specific tissue components such as connective tissue septa or immune cell infiltrates in liver tissue. This process may reveal new biological properties associated with specific diseases or clinical courses and thus fundamentally expand our understanding of liver diseases.
4.) The development of a user-friendly and intuitive online platform for the broad dissemination of the developed methods and their systematic evaluation.
The long-term goal of our consortium is to introduce novel diagnostic and prognostic methods into mainstream medical practice. We aim to develop a prototype web application in which registered professionals can enter patient data and scanned histological images in anonymised form, which will then be automatically analysed. Based on this, a structured report is created within a few minutes and made available to the user.
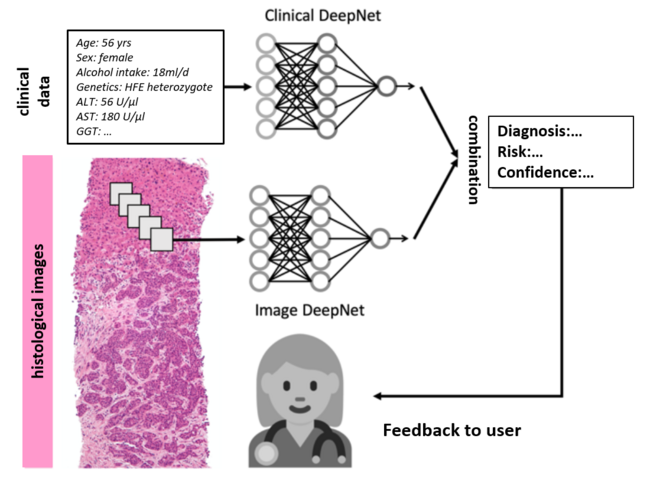